Uplift Bank Profits. AI to Maximize Customer Lifetime Value (Product Holding Ratio). (LLM Part 13)

The postings on this site are my own and do not necessarily represent the postings, strategies or opinions of my employer.
- On LLM Series.
- On Cloud Series.
- On Platformization of Banking Series.
- AI and Data: The Powerhouse for Banks
- Reimagining Recommendations: LLMs as a New Frontier (LLM Part 12)
- Uplift Bank Profits. AI to Maximize Customer Lifetime Value (Product Holding Ratio). (LLM Part 13)
On this post we cover:
- Basic introduction
- AI framework for banks
- The Generative AI Value-Creation Framework
- CLV (Product Holding Ratio) in Banking
- AI Agent - Customer Lifetime Orchestrator solution of Backbase
- Generative AI Tools for SMEs
- Capitalizing on Data - "single source of truth" (SSOT) and "multiple versions of the truth" (MVOTs)
Introduction
In today's competitive banking landscape, maximizing profitability is paramount. While traditional metrics like quarterly earnings remain important, a more strategic approach focuses on the long-term value of customer relationships. This is where Customer Lifetime Value (CLV) comes into play. CLV represents the total revenue a bank expects to generate from a single customer throughout their relationship or simply we refer to it product holding ratio.
By understanding and maximizing CLV (product holding ratio), banks can shift from short-term gains to sustainable growth. However, achieving this requires effectively analyzing vast amounts of customer data and predicting future behavior – a challenge perfectly suited for the power of AI. This blog post will explore how AI can revolutionize CLV (product holding ratio) maximization in the banking sector, driving increased profits and fostering stronger customer relationships.
We are witnessing one of the most significant platform shifts in history. As the personal computer democratized computing power and the internet revolutionized distribution, generative AI is ushering in an era of dramatically reduced productivity costs.
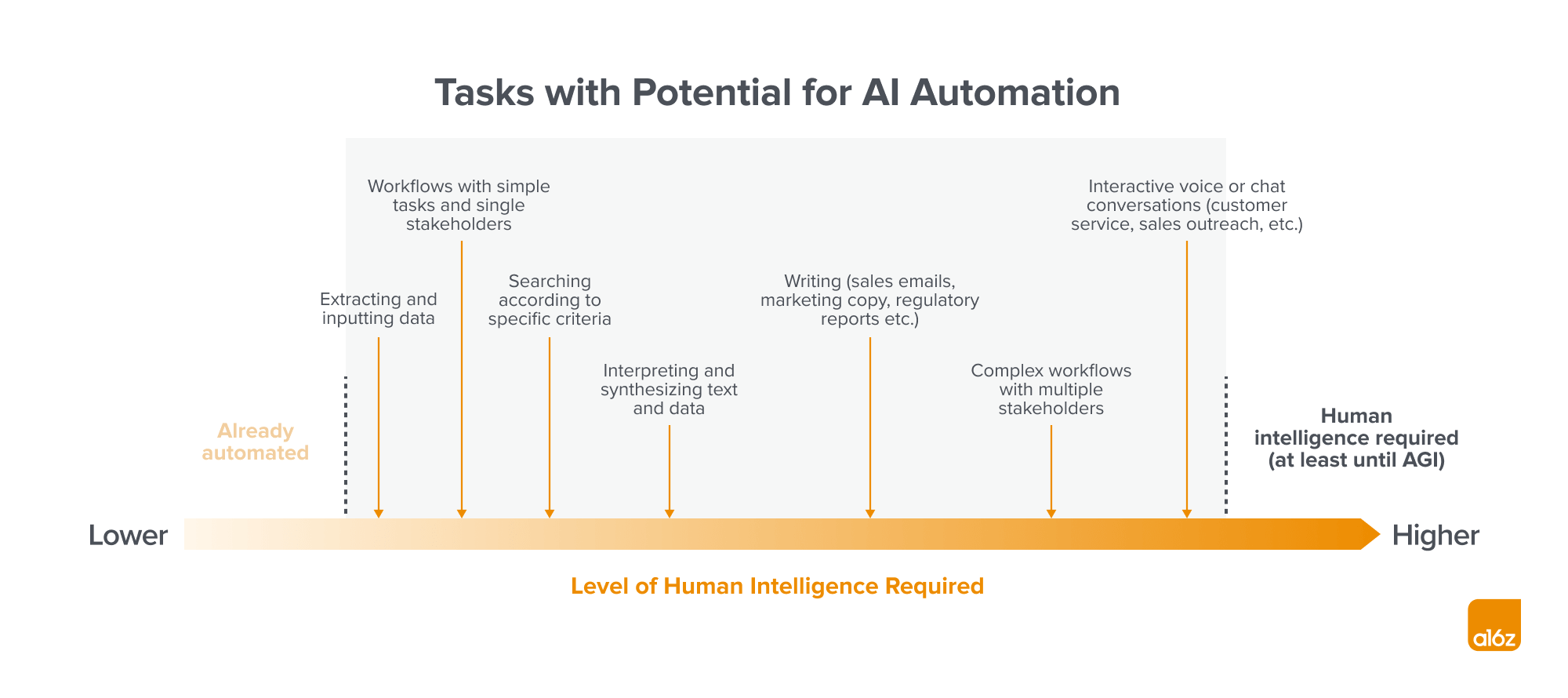
Jobs largely defined by the tasks outlined above face near-total automation by AI agents. For example:
Traditional Know Your Business (KYB) processes are often bogged down by manual tasks. For instance, analysts might verify a business address by searching Google Maps and comparing it to a description. Luckily, this is where AI shines. Tools like Parcha or Greenlite can automate much of this legwork, freeing up human analysts. Similarly, platforms like Norm.ai utilize AI to ensure marketing copy adheres to regulations like the SEC marketing rule, replacing the need for tedious human review.
Sales development reps (SDRs): Sales teams spend much of their time searching for and qualifying leads, inputting data, drafting outreach, and managing pipeline reports — rather than actually selling. New players like 11x are automating these tasks, so sales teams can spend more time on high value work and face time with customers.
Loan Origination Systems (LOS) are becoming increasingly automated. For example, Vesta, used for mortgages, automatically ingests documents, accurately extracts key data, and even communicates with borrowers to request missing paperwork. Casca, designed for commercial loans, claims to boost productivity tenfold and reduce manual work by 90%.
AI Framework for Banks
This article from McKinsey & Company provides a comprehensive framework for banks to adopt AI and transform their operations. It's a reset time (again). AI can be integrated into various aspects of banking, from customer engagement to risk management. It highlights the importance of a strong technological foundation, including data infrastructure, machine learning operations, and security. Additionally, it emphasizes the need for a cultural shift towards AI-driven decision-making and agile ways of working. By embracing AI and rethinking their technology stack, banks can deliver exceptional customer product holding, improve CIR, and gain a competitive edge.
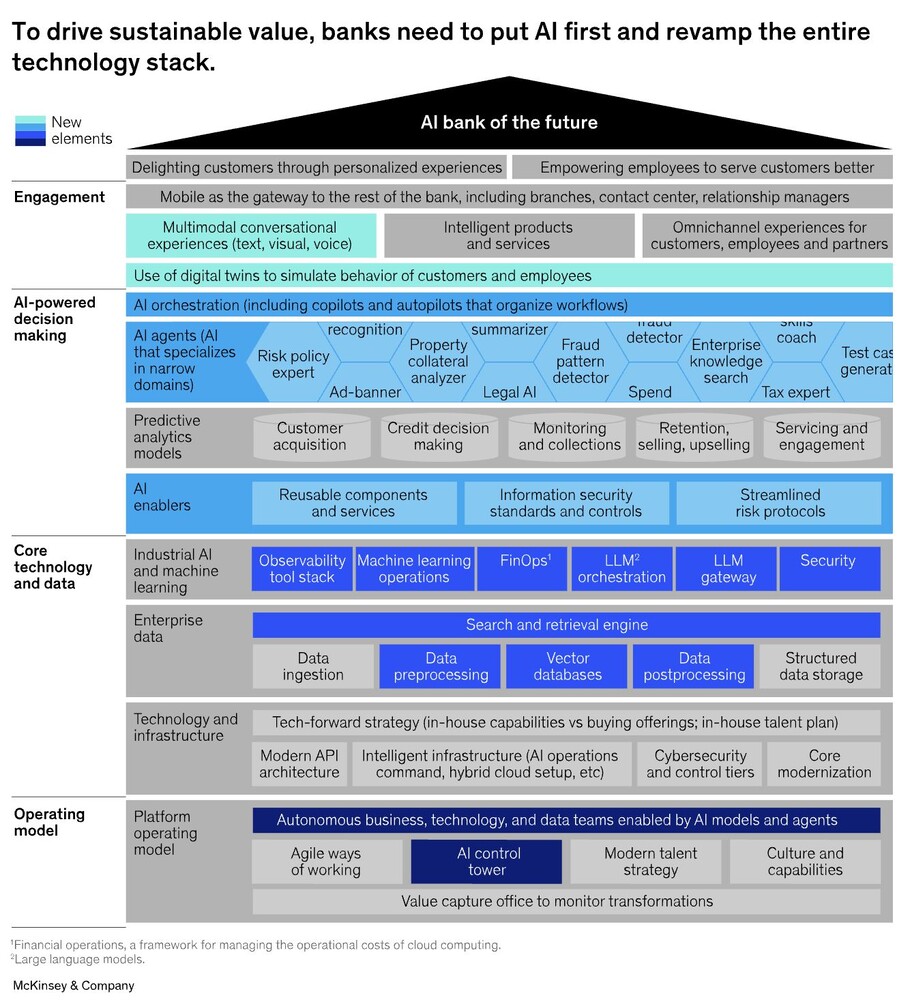
The Generative AI Value-Creation Framework
The key difference between generative AI that delivers incremental gains and that which drives transformative change lies not in technical prowess or strategic foresight alone, but in a deep understanding of your organization’s performance drivers. This framework offers a clear path to assess generative AI maturity and build the necessary capabilities to unlock its full potential. While initial efforts often focus on boosting individual productivity, market leaders progress through four levels of competence to systematically create value: individual improvements, collective intelligence, transformation & growth, and visionary innovation.

Note that each level builds upon previous capabilities. Let’s walk through each one.
- Individual Improvements. Individual use of generative AI can lead to significant productivity gains for specific tasks, as shown by studies of customer service agents, software engineers, and data scientists. However, these individual improvements, while substantial (e.g., up to 34% faster issue resolution for customer service), translate to a much smaller overall impact at the enterprise level. This limited impact aligns with the concept of "so-so technologies," which displace workers without significantly boosting overall productivity or competitiveness. Many organizations are currently at this stage, simply providing access to generative AI without a broader strategy, resulting in minimal overall effect and potentially even falling behind competitors.
- Collective Intelligence. Effective teamwork relies on shared understanding. Organizations using generative AI to bridge communication gaps and foster consensus gain a competitive edge. Research links shared understanding of work purpose and context to better output. Generative AI helps teams overcome collaboration barriers, identify shared mental models, reduce bias, and resolve conflicts faster. For example, an insurer used generative AI to clarify task definitions and improve productivity after a merger. The key is not just AI usage, but establishing a shared understanding of its capabilities, treating it as a specialized team member collaborating with human experts to unlock deeper potential and achieve significant results.
- Transformation & Growth. While individual productivity gains are useful, generative AI's true potential lies in fundamentally changing how work is done, creating entirely new value sources. For example, AI can handle documentation for doctors, allowing for more focused patient interaction. The Cleveland Clinic is implementing AI to reduce paperwork and increase patient care time. However, using AI for critical tasks requires strong ethical and safety protocols, as emphasized by the WHO. The Cleveland Clinic prioritizes human oversight alongside AI implementation. Organizations should balance innovation with responsibility by creating dedicated experimentation spaces to test new approaches and discover novel ways to augment expertise with generative AI.
- Visionary Innovation. After reimagining internal processes with generative AI, organizations can transform external interactions with customers and stakeholders, creating innovative products and services. Research shows generative AI can accelerate innovation (e.g., increased material discovery and patent filings), but can also decrease job satisfaction due to perceived loss of creativity. Human skills training can mitigate this. Organizations should focus on high-impact experiences and scalable efficiencies to drive customer value. For example, a distributor used a custom GPT to generate customer personas, revealing marketing blind spots and sparking new thinking about customer interactions. This ignited further innovation across business units, leading to new AI-driven products and services.
CLV (Product Holding Ratio) in Banking
The traditional banking model of attracting customers and deepening the relationship and increasing product holding ratio (ultimately CLV) through extensive branch networks is becoming obsolete. The shift to electronic transactions and the emergence of digitally focused competitors necessitate a digital-first strategy for driving CLV. Banks that prioritize a mobile-first, omnichannel approach to acquiring, activating, deepening, and retaining these relationships will be better equipped to boost net interest margin (NIM) and fee revenue while lowering costs, potentially achieving cost-to-income ratios (CIRs) of 40–50% or lower.
Digital technology has fundamentally altered how banks establish and maintain product holding ratio. Banks must shift their focus to robust digital capabilities and seamless cross-channel integration, using assisted channels to enhance the digital experience. However, this new paradigm presents significant hurdles:
- Acquisition and Account Opening: Banks must enhance digital marketing and develop new skills to attract high-quality digital traffic, avoiding costly low-value acquisitions. Customers expect faster, more streamlined account opening, but the lack of face-to-face interaction requires banks to find new ways to build customer connection and brand affinity.
- Activation: Encouraging active usage of digitally opened accounts is a significant hurdle. Due to lower initial commitment, many customers fail to develop regular banking habits, resulting in activation rates of only 30-40% within the first 90-180 days.
- Deepening: Customers typically divide their banking relationships across multiple banks, holding only a few products with their main bank. Digital channels offer frequent engagement opportunities, but banks should prioritize building trust and understanding customer needs over direct selling. Personalized, timely communication (with frequent refinement) and integration with human interaction are essential. Offering easily accessible digital products and third-party services is also crucial.
- Retention: Data analytics is crucial for identifying and addressing declining customer engagement. Increased transparency regarding service issues across all touchpoints is also essential for effective retention strategies.
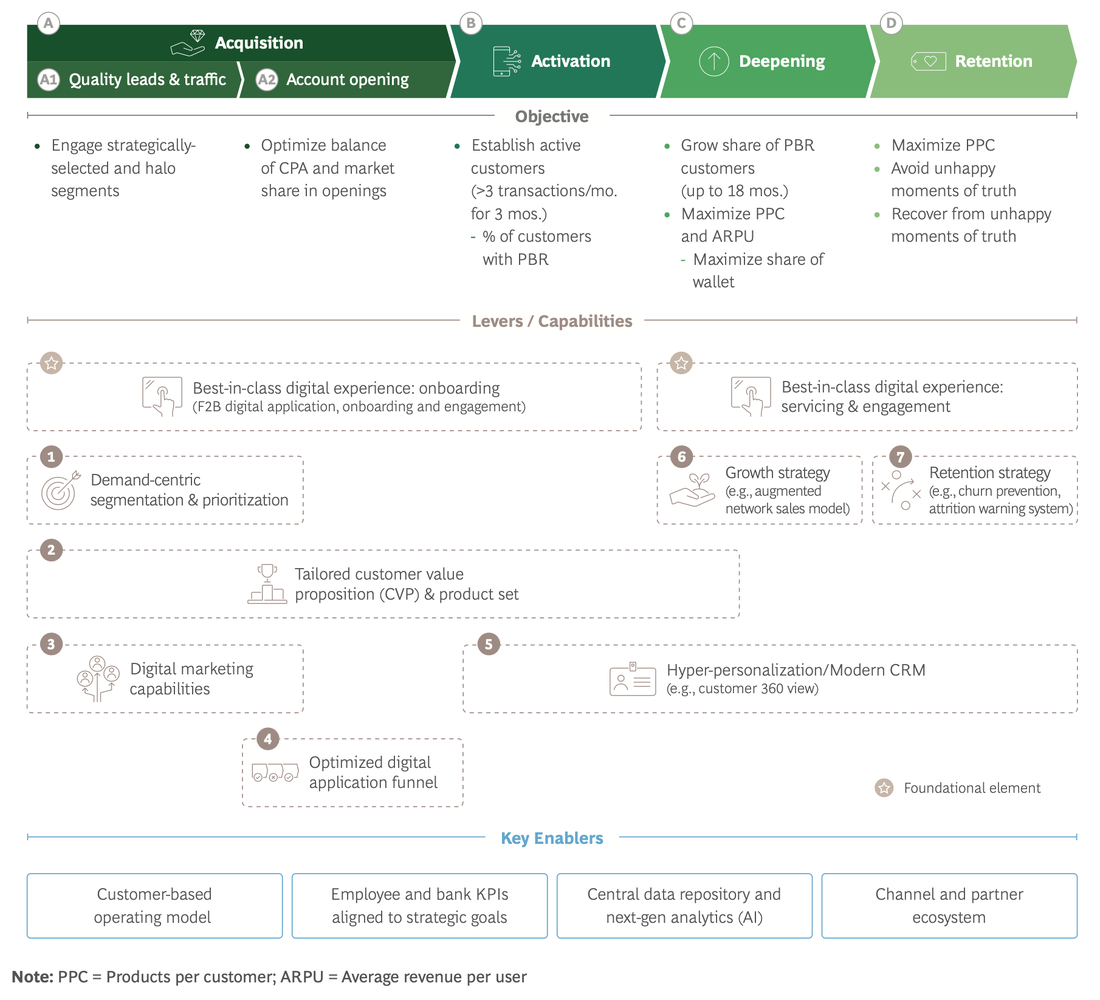
Here are examples of how AI can address the challenges banks face in acquisition and account opening, activation, deepening, and retention:
Acquisition and Account Opening:
- AI-powered lead scoring: AI algorithms can analyze vast datasets (including demographics, online behavior, and social media activity) to identify high-potential leads, allowing banks to focus their marketing efforts on the most promising prospects. This reduces wasted ad spend on low-quality traffic.
- Automated KYC/AML (Know Your Customer/Anti-Money Laundering): AI can automate identity verification, document processing, and risk assessment, streamlining the account opening process and reducing manual effort. This leads to faster onboarding and improved customer experience.
- AI-driven chatbots for onboarding: Chatbots can guide new customers through the account opening process, answering questions, providing support, and collecting necessary information. This provides 24/7 availability and reduces customer wait times.
- Personalized onboarding experiences: AI can personalize the onboarding journey based on individual customer profiles and needs. For example, offering tailored product recommendations or educational content related to specific financial goals.
Activation:
- AI-driven personalized product recommendations: By analyzing customer data and behavior, AI can recommend relevant products and services to newly acquired customers, encouraging them to start using their accounts.
- Proactive engagement through AI-powered chatbots or virtual assistants: AI can proactively engage with new customers through personalized messages, offering assistance with account setup, explaining key features, and providing relevant financial tips.
- Gamification and incentives driven by AI: AI can personalize gamified experiences and offer targeted incentives to encourage account usage. For example, offering rewards for completing specific actions, such as setting up direct deposit or making a first transaction.
- Predictive analytics for early churn detection: AI can identify early indicators of potential churn among new customers, allowing banks to intervene with targeted campaigns or personalized offers to re-engage them.
Deepening:
- AI-powered personalized financial advice: AI can analyze customer transaction data, spending habits, and financial goals to provide tailored financial advice, helping customers manage their finances more effectively and increasing engagement with the bank.
- AI-driven cross-selling and upselling: AI can identify opportunities to offer relevant products and services to existing customers based on their individual needs and preferences. This increases product penetration and revenue.
- Personalized content and offers: AI can deliver targeted content and offers through various channels (email, mobile app, website) based on individual customer profiles and behavior. This improves engagement and strengthens customer relationships.
- AI-powered chatbots for customer service and support: Chatbots can provide 24/7 customer support, answering questions, resolving issues, and providing personalized assistance. This improves customer satisfaction and frees up human agents to handle more complex issues.
Retention:
- Predictive analytics for churn prevention: AI can identify customers at high risk of churning by analyzing various data points, such as transaction history, online behavior, and customer service interactions. This allows banks to proactively engage with these customers and offer personalized incentives to retain them.
- AI-powered sentiment analysis: AI can analyze customer feedback from various sources (surveys, social media, online reviews) to identify negative sentiment and address potential issues before they lead to churn.
- Personalized retention offers and campaigns: AI can personalize retention offers and campaigns based on individual customer profiles and needs. This increases the effectiveness of retention efforts and improves customer loyalty.
- AI-driven customer service recovery: When a customer experiences a negative interaction, AI can help identify the issue and trigger automated or personalized recovery actions. This can help rebuild customer trust and prevent churn.
Amsterdam, 25 September 2024 - Backbase is excited to announce a major expansion of the Engagement Banking Platform with the introduction of the Intelligence Fabric layer, a powerful set of data and AI infrastructure and development capabilities that are now natively embedded in the platform. This groundbreaking launch is set to redefine how banks can harness the power of data and AI to unlock significant productivity gains within their business-critical customer servicing and sales operations.
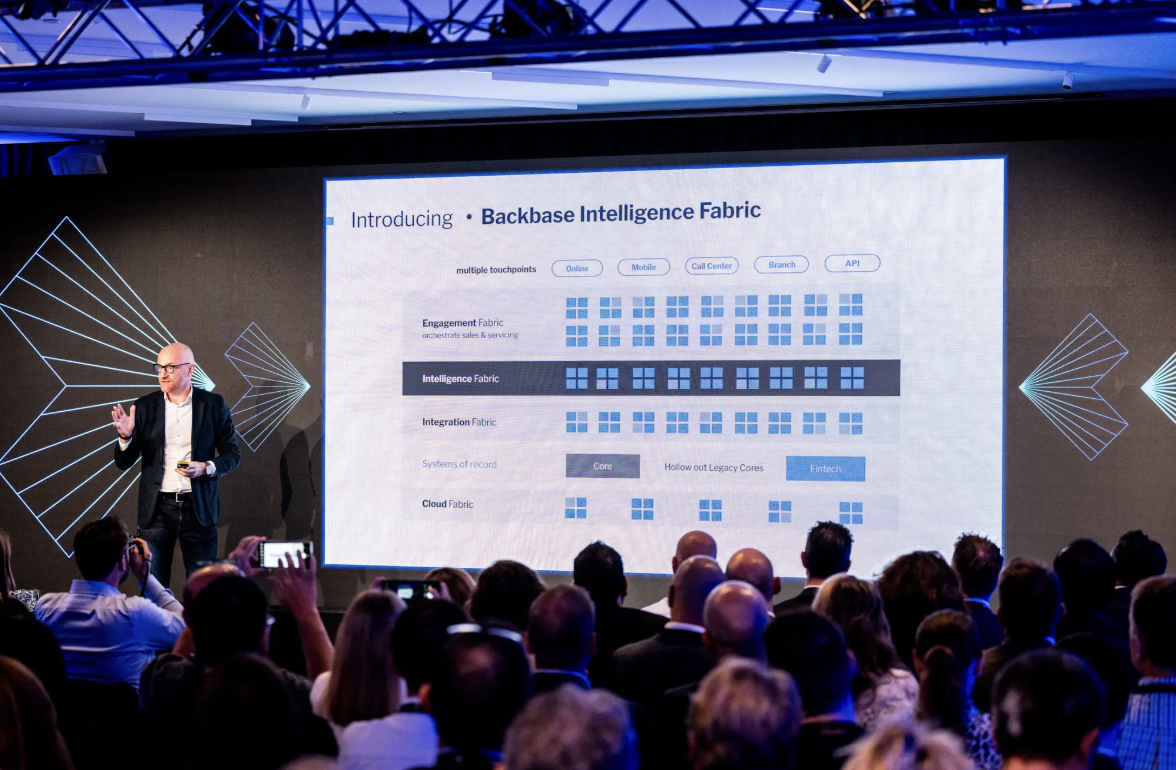
AI is already playing a pivotal role in transforming banking, driving efficiency, improving risk management, and orchestrating contextual, relevant customer experiences. As the AI super-cycle continues to reshape the financial landscape, Backbase is rising to meet these challenges head-on with the introduction of the Intelligence Fabric.
Jouk Pleiter , Founder and CEO at Backbase, commented,“Backbase is leading the way with its AI platform capabilities for banking. The introduction of the Intelligence Fabric marks a pivotal moment in our mission to empower banks to harness the power of data and AI at scale. Today, we are making a massive leap forward in unveiling our Agentic AI strategy. We see a future where AI Agents will work autonomously in the background, handling tasks, managing processes, and collaborating with customers and employees. The adoption and evolution of these new-gen, super-powerful agents will dramatically reduce internal and external labor spend on overheads such as sales, marketing, customer service, and compliance operations.”
Backbase is prioritizing the availability of AI-Agent capabilities for the following use cases:
- Conversational Banking: leverage large language models (LLMs) to handle daily banking tasks like accessing accounts, making payments, checking transaction histories, and managing cards across digital channels.
- Customer Lifetime Orchestration: create AI-driven product activation and up-sell campaigns that enable the bank to increase the product holding per customer. AI-driven predictive nudges will provide contextual guidance to customers, promoting relevant new products such as credit cards, loans, savings, investing, and insurance, all based on customer behavior and financial history.
Episode 21 of Banking Reinvented focuses on the strategies banks can adopt to grow revenue, particularly by optimizing customer relationships through personalization and AI. Host Tim Rutten is once again joined by Thomas Fuss, CTO at Backbase, to discuss the challenges in the traditional banking model, where the focus often stops at customer acquisition. They explore the concept of customer lifecycle orchestration and the importance of breaking down organizational silos to deliver personalized services that meet evolving customer needs.
AI Agent - Customer Lifetime Orchestrator solution of Backbase
Our newest release empowers your bank to harness the power of AI and unified data, driving substantial productivity gains across your most vital customer service, sales, and operational workflows. With the Intelligence Fabric, your bank can seamlessly create AI Agents that augment and orchestrate every customer journey. These agents work hand-in-hand with your existing workflows, integrations, and real-time data. By unifying enterprise-wide data, tools, and processes on the Engagement Banking Platform, they interpret contextual information to deliver personalized, timely responses — empowering you to elevate customer engagement at every touchpoint.
Redefine how your bank drives growth with intelligent systems that enhance efficiency, elevate risk management, and orchestrate contextual, personalized customer experiences — all at scale.
Conversational banking - Transform your customer interactions
Allow your customers to leverage large language models (LLMs) to handle daily banking tasks, including accessing accounts, making payments, checking transaction histories, and managing cards across digital channels.
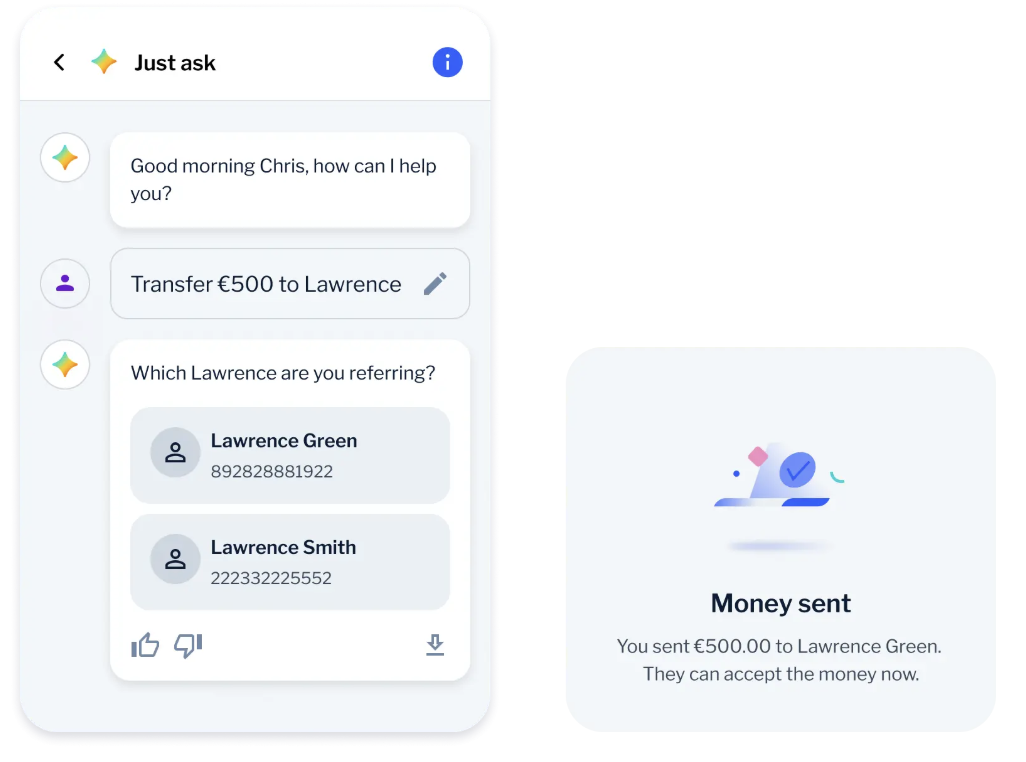
Customer Lifetime Orchestrator (CLO)
Create AI-driven product activation and up-sell campaigns across a timeline that will enable your bank to increase product holding per new-to-bank and existing customer. Predictive nudges provide contextual guidance to customers, promoting relevant new products such as credit cards, loans, savings, investments, and insurance, all based on behavior and financial history.

Generative AI Tools for SMEs
Generative AI is transforming the landscape for small and medium-sized enterprises (SMEs) as well, providing access to tools that were once exclusively available to larger corporations. This is particularly evident in marketing, where content creation has traditionally been a significant hurdle. Now, with generative AI, SMEs can easily and affordably produce high-quality product descriptions, visuals, and advertisements. Platforms like Jasper streamline marketing content creation, while Canva and Adobe Firefly democratize access to professional-grade graphic design. While these platforms tout their enterprise clients, the real impact is felt by SMEs. User reviews highlight the transformative effect: a musician launching their career, an immigrant software engineer communicating effectively, and small businesses achieving professional SEO. The overwhelmingly positive feedback from SMEs using Neuroflash further underscores this trend.
The growing availability of open-source text and image generation models is a significant development, making large language models (LLMs) more affordable and adaptable. Mistral AI, for example, offers a free, downloadable LLM that can run on standard PCs, eliminating the need for costly hardware. These open-source models can also be tailored to specific business needs. A recent study demonstrated this by developing an open-source AI-based ad generation algorithm that not only preserved brand personality but also outperformed existing industry ads. Generative AI's capabilities extend beyond text and images. Google's MusicLM generates music from text prompts (e.g., "the main soundtrack of an arcade game"), and OpenAI has released an open-source music composition algorithm, including vocal tracks. AI-generated music is already being used in advertising, particularly for perfumes and cars. While these advancements benefit large companies, they offer even greater value to SMEs, which often struggle with the high cost of music royalties, providing them with access to affordable, high-quality musical content.
AI provides SMEs with powerful analytical tools, generating market and customer insights from large datasets. Tools like OpenAI's Code Interpreter allow even non-experts to analyze data and create visualizations using simple prompts. Generative AI can also simulate human data. While research suggests LLM outputs may be biased towards WEIRD societies, this depends on the specific model and prompts. For instance, GPT-4 has shown the ability to accurately reflect cultural differences when prompted appropriately.
All in all, multi-modal and multi-agent generative AI has the potential to close the content, insight, and technology gaps that large corporations typically have over their smaller counterparts.
Capitalizing on Data - "single source of truth" (SSOT) and "multiple versions of the truth" (MVOTs)
Unlocking the full value of data is becoming a key competitive advantage, making data management, governance, analysis, and security a crucial new business function. Combining data from multiple sources (e.g., sensor readings from various car components) can reveal valuable insights and predict potential problems (like impending bearing failure). This is the domain of big data analytics, which uses mathematical, computational, and business analysis techniques. Because this data is often unstructured and in various formats, traditional analysis methods are insufficient. The solution is a "data lake," a repository for storing diverse data in its native formats, which can then be analyzed using descriptive, diagnostic, predictive, and prescriptive analytics tools.
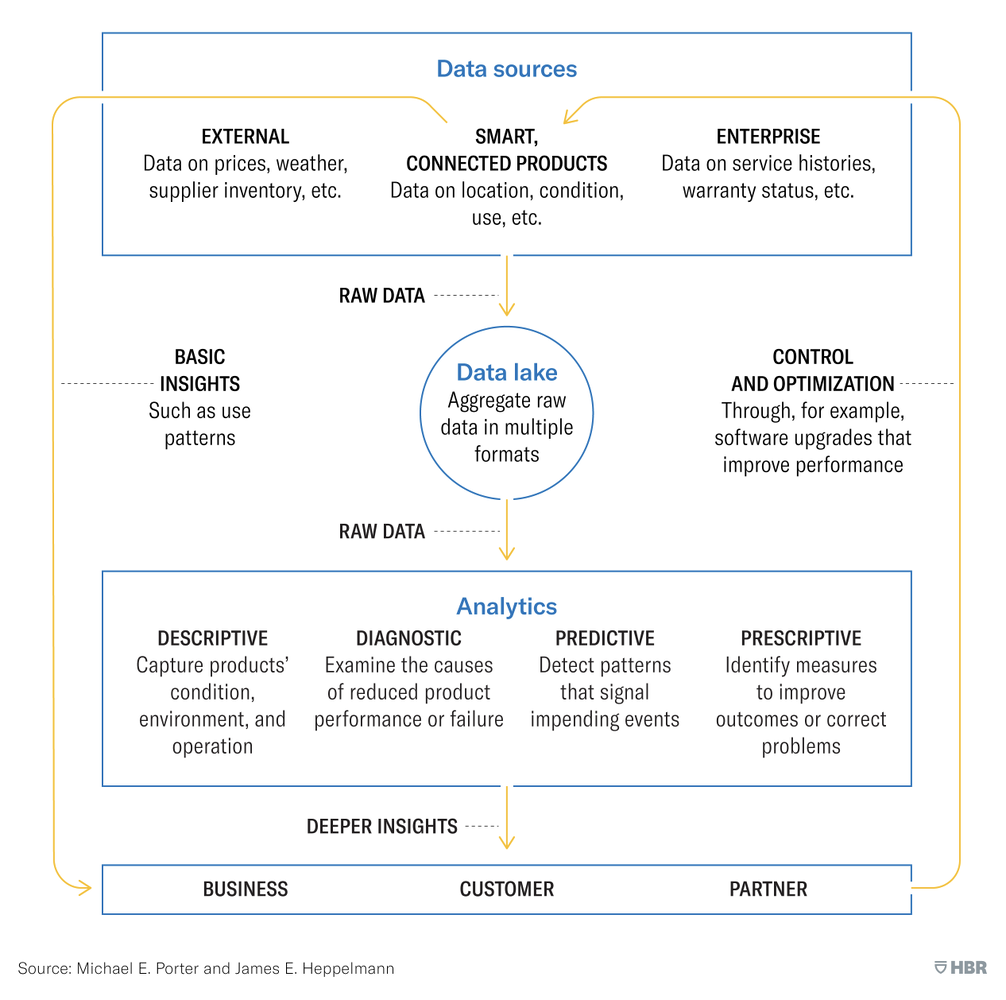
This framework focuses on two key aspects of data strategy: clarifying the primary purpose of data and guiding strategic data management. It distinguishes between "defensive" and "offensive" uses of data, requiring companies to make strategic trade-offs between these approaches and between control and flexibility.
Unlike purely technical data management frameworks, this business-focused approach aligns data activities with overall business strategy, emphasizing efficient resource allocation.
Data defense aims to minimize downside risks, focusing on compliance (e.g., data privacy), fraud detection, and system security. It also ensures data integrity by establishing a "single source of truth" for core data like customer information and sales data. Data offense, conversely, supports business growth objectives like increasing revenue and customer satisfaction. This involves generating customer insights through data analysis, modeling, and integrating diverse data sources to support managerial decisions through tools like interactive dashboards.
Offensive activities are typically more relevant to customer-facing functions like sales and marketing and often operate in real-time, unlike defensive activities, which are more focused on legal, financial, compliance, and IT concerns (with the exception of real-time fraud detection).
Balancing these competing demands for resources is crucial. While some companies may benefit from equal emphasis on both, others should prioritize either defense (e.g., in highly regulated industries) or offense (e.g., in highly competitive markets) to align with their overall strategic goals. This balance also requires finding equilibrium between data standardization (for defense) and flexibility (for offense). The framework emphasizes the importance of both a "single source of truth" (SSOT) at the data level and "multiple versions of the truth" (MVOTs) at the information level to support both defensive and offensive strategies.
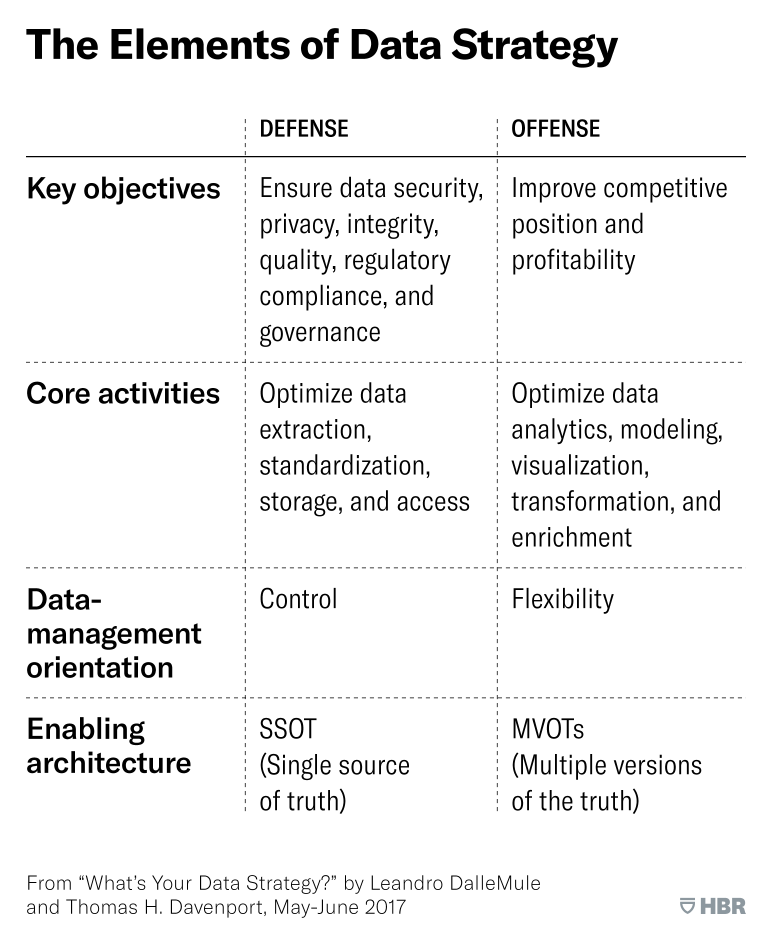
The Single Source of Truth (SSOT) is a central, often cloud-based repository containing a single authoritative copy of critical data like customer, supplier, and product details. It requires strong data governance and provenance to ensure reliability for both defensive and offensive data use, using a common, consistent language across all business units. Without an SSOT, data inconsistencies arise, as exemplified by a company with multiple, conflicting sources of supplier information. This makes it difficult for traditional systems to understand key relationships. AI tools are now available to create SSOTs from disparate data, saving costs by eliminating redundant systems. In one case, implementing an SSOT allowed a company to identify multi-unit suppliers and negotiate discounts, resulting in $75 million in first-year benefits.
The Single Source of Truth (SSOT) serves as the foundation for creating Multiple Versions of the Truth (MVOTs). MVOTs are created by transforming raw data from the SSOT into relevant and purposeful information for specific business needs. Different groups within an organization can create distinct, controlled MVOTs that provide consistent, customized responses based on their requirements. For example, a supplier might classify clients like Bayer and Apple broadly at the SSOT level (pharmaceuticals and consumer electronics, respectively), but sales teams might use more granular classifications (mobile phone company or drug company) for better decision-making.
The key is that these MVOTs are derived from a common SSOT, ensuring consistency and traceability. This allows for superior decision-making by providing tailored information to different parts of the organization. For instance, a financial company's marketing and finance departments might report different figures for ad spending (based on airtime vs. payment date), both accurately reflecting their specific needs.
Companies like Procter & Gamble have also adopted this approach. While they maintain a centralized SSOT, they now allow business units to create controlled, traceable transformations of that data for their specific reporting needs, recognizing the value of customized interpretations. This approach allows for both consistency (through the SSOT) and flexibility (through MVOTs), supporting a more effective data strategy.
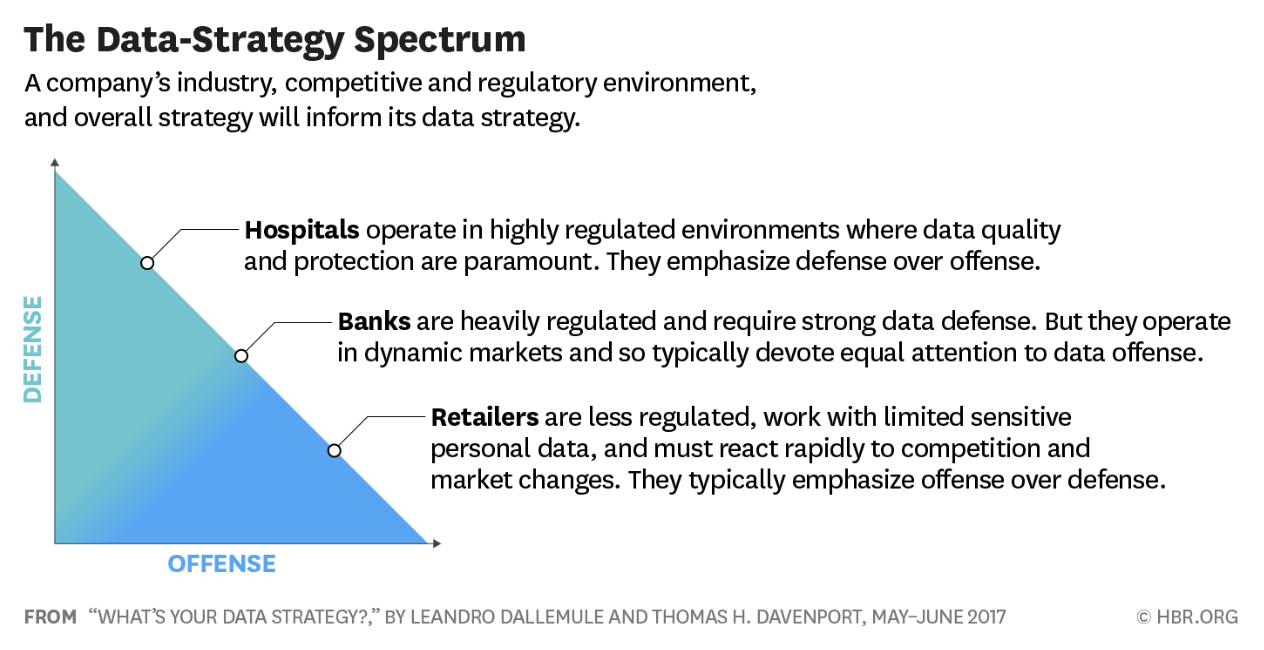
The Canadian Imperial Bank of Commerce (CIBC) uses the SSOT-MVOTs model, automating processes to maintain alignment between source data and transformed data. Their SSOT holds core client data, while MVOTs for loan origination and CRM adapt this data for regulatory reporting and enhanced customer experience. Automated synchronization and nightly "exception handling" ensure data integrity. While the SSOT-MVOTs model is conceptually simple, it demands strong data controls, standards, governance, and technology, ideally with senior executive involvement. Though often led by CDOs and CTOs with participation from business and technology managers, the key is maintaining the uniqueness and validity of the SSOT and ensuring controlled divergence in MVOTs