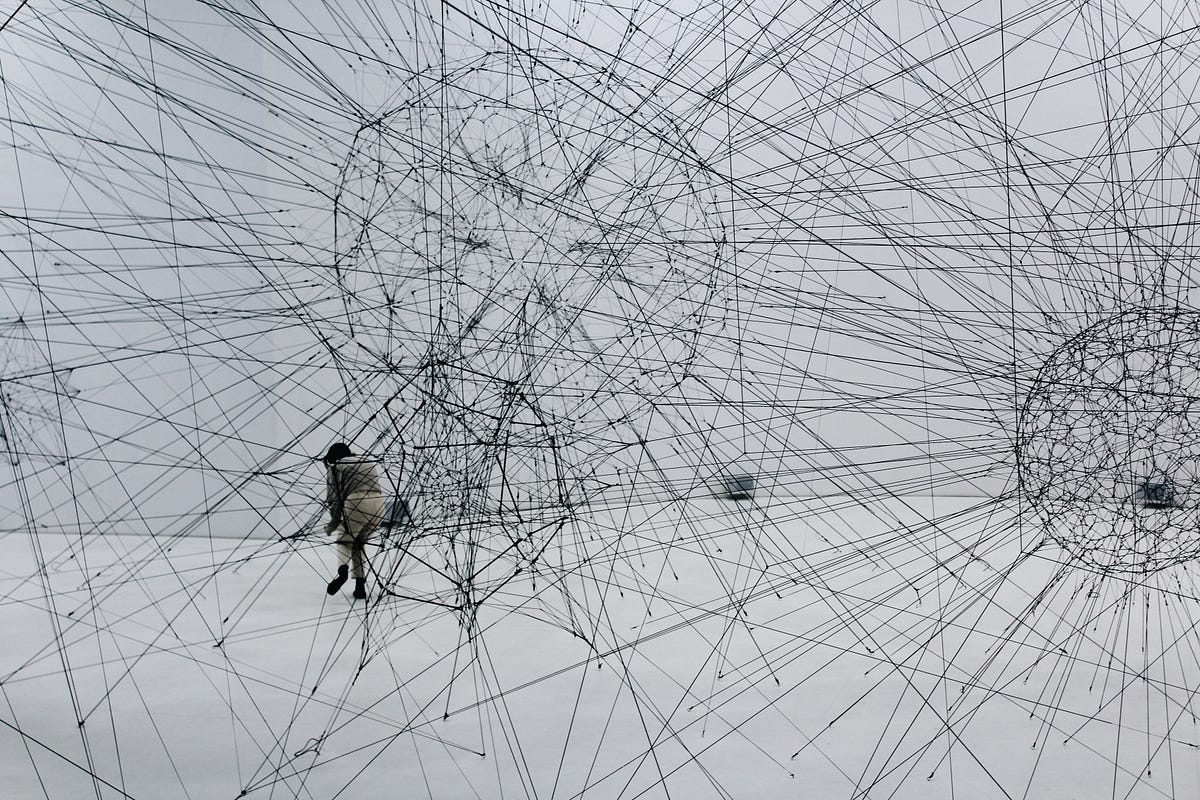
The postings on this site are my own and do not necessarily represent the postings, strategies or opinions of my employer.
- On LLM Series.
- On Cloud Series.
- On Platformization of Banking Series.
- AI and Data: The Powerhouse for Banks
- Reimagining Recommendations: LLMs as a New Frontier (LLM Part 12)
- Uplift Bank Profits. AI to Maximize Customer Lifetime Value (Product Holding Ratio). (LLM Part 13)
- AI-Driven Customer Lifetime Orchestration for Banks (LLM Part 14) - This is co-authored with Quynh Chi Pham and Amelia Longhurst .
- Augmented Intelligence (LLM Part 15) in banking
- Data Mesh in Banking. Orchestrating CLV. (LLM Part 16)
Banks today face a dual challenge: managing ever-increasing volumes of data while simultaneously trying to leverage that data for cutting-edge AI initiatives. Traditional data architectures often fall short, creating data silos and hindering agility. Imagine a bank where data flows seamlessly, empowering data scientists to build and deploy AI models with unprecedented speed and efficiency. Imagine a bank where data governance is streamlined, ensuring compliance and trust. This vision can become a reality with Data MeshThis vision can become a reality with Data Mesh, enabling banks to overcome these hurdles and build a truly data-driven future. Explore the table of contents below to learn how Data Mesh can revolutionize your data strategy and unlock the power of AI.
- The Data & AI Imperative in Banking: Driving Business Value in a Competitive Landscape
- Understanding Data Mesh: Core Principles for AI-Ready Data in Banking
- Lessons for a New Data Architecture
- JPMorgan Chase & Fannie Mae run a data mesh
- Unlocking Business Value with Data Mesh and AI: A CDO/Head of Data Science Perspective in Banking
- Data Mesh Implementation in Banking: Key Considerations for AI Success
- Orchestrating Customer Value: The Role of CLO in the Banking Data Mesh
- The Future of Banking: Data Mesh, AI, and the Rise of the Data-Driven Bank
The Data & AI Imperative in Banking: Driving Business Value in a Competitive LandscapeThe banking industry is at a critical juncture. Disruptors are rapidly reshaping the financial landscape, forcing traditional institutions to adapt or risk being left behind. Customer expectations are soaring, demanding personalized experiences and seamless digital interactions. Simultaneously, regulatory scrutiny is intensifying, requiring banks to maintain strict compliance and manage risk effectively. In this environment, data and AI have emerged as crucial differentiators, offering banks the opportunity to not only survive but thrive. The potential benefits of effective data and AI strategies for banks are substantial. Personalized customer offers, tailored financial advice, and proactive customer service can significantly boost customer satisfaction and loyalty, leading to increased revenue and market share. By leveraging data and AI effectively, banks can unlock new revenue streams, reduce operational costs, and gain a significant competitive edge. However, banks face unique challenges in leveraging data for AI. Legacy systems often create data silos, making it difficult to access and integrate information across different departments. Regulatory constraints and customer privacy concerns require careful handling of sensitive data. Furthermore, a shortage of skilled data scientists and AI specialists can hinder the development and deployment of AI solutions. Data Mesh offers a powerful solution to these banking-specific challenges. By decentralizing data ownership and treating data as a product, Data Mesh empowers banks to break down data silos, improve data quality, and accelerate AI adoption. Within this framework, tools like Customer Lifetime Orchestrator (CLO) become essential, enabling banks to leverage data insights for personalized customer relationship management and maximize customer lifetime value. Understanding Data Mesh: Core Principles for AI-Ready Data in BankingData fabric and data mesh are independent concepts that can coexistThe terms “data fabric” and “data mesh” are often used interchangeably or even discussed as competing approaches. In fact, they are independent concepts. Under the right circumstances, they can be used to complement each other. To recap, data fabric is an emerging data management design that uses metadata to automate data management tasks and eliminate manual data integration tasks. Data mesh, in contrast, is an architectural approach with the goal of building business-focused data products in environments with distributed data management and data governance responsibilities. Though data fabrics are about data management and data mesh is about data architecture, both share the same goal of enabling easier access to and use of data. Some key distinctions and complements are:
- Technology — A data fabric can work with different integration styles in combination to enable a metadata-driven implementation and design. A data mesh is a solution architecture that can guide design within a technology-agnostic framework.
- Purpose — A data fabric discovers data optimization opportunities through the continuous use and reuse of metadata. A data mesh takes advantage of business subject matter expertise to develop context-based data product designs.
- Data authority and data governance — A data fabric recognizes and tracks data use cases that can be authoritative, and it treats all subsequent reuse by adding to, refining and resolving data authority differently by use case. A data mesh emphasizes the originating data sources and use cases to produce combinatorial data products for specific business contexts.
- People — A data fabric encourages augmented data management and cross-platform orchestration to minimize human efforts. A data mesh, at present, promotes the ongoing manual design and orchestration of existing systems with human intervention during maintenance.

Data Mesh represents a paradigm shift in how banks manage and utilize data, particularly in the context of AI.
Data Mesh is a decentralized approach that treats data as a product, empowering domain teams to own and manage their data while adhering to overarching governance principles.
This approach stands in contrast to traditional centralized data architectures, which often struggle to keep pace with the volume, velocity, and variety of data needed for modern AI applications in banking. Data Mesh is built on four core principles, each crucial for creating AI-ready data.
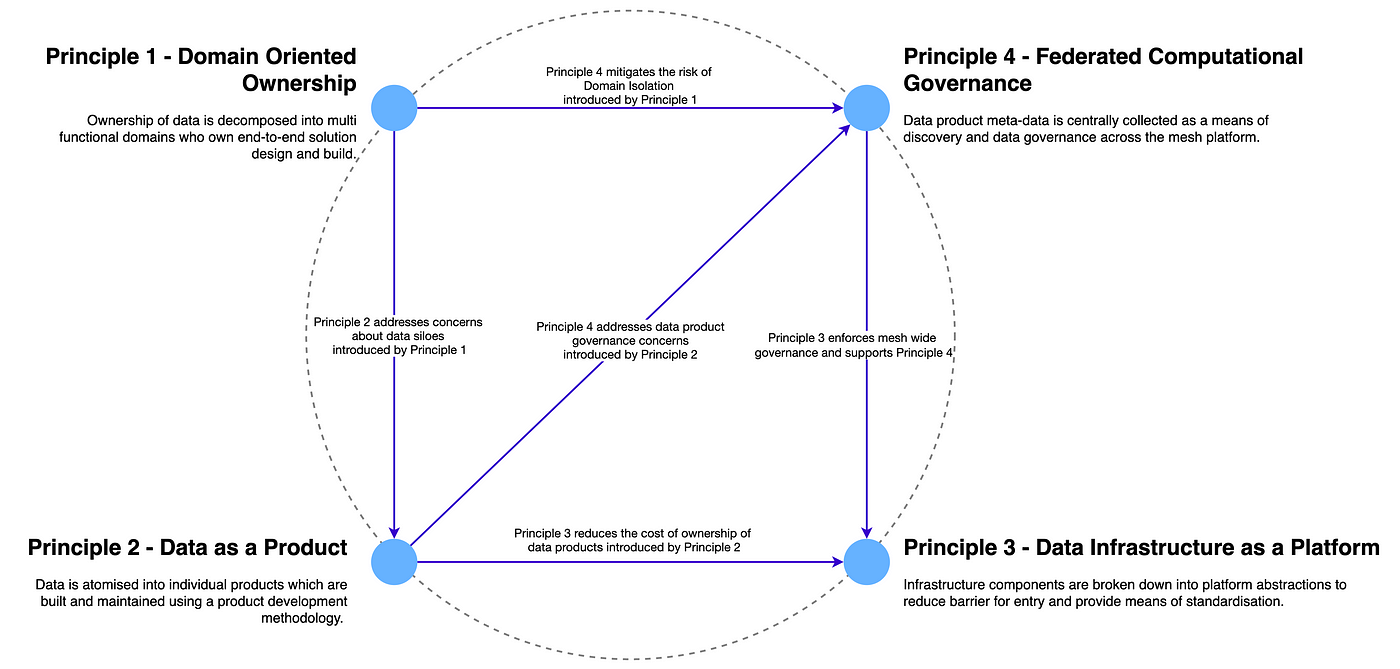
Principle #1 - Domain Centricity
The first principle is decentralized data ownership, also known as domain-centricity. In a bank, this means that different departments or business domains own and manage the data relevant to their operations. For example, the lending department would own and manage loan data, while the retail banking division would be responsible for customer data. This decentralized ownership is critical for AI because domain teams have the deepest understanding of their data, including its nuances, quality, and relevance to specific AI use cases. By empowering domain teams, banks can improve the accuracy and effectiveness of AI models for tasks like loan approvals or personalized offers, as these models are trained on data managed by those closest to it.

Principle #2 - Data as a Product
The second principle is data as a product. This means treating data not as a mere byproduct of operations but as a valuable asset with its own lifecycle. A "Customer 360" data product, for instance, might contain a comprehensive view of a customer, including transaction history, demographics, risk scores, and product holdings. This data product would be designed specifically for AI-driven personalization, enabling the bank to offer tailored financial advice or targeted marketing campaigns. By treating data as a product, banks ensure that it is well-documented, easily discoverable, and of high quality, making it readily available for AI/ML teams.

Principle #3 - Self-service Data Infrastructure
The third principle is self-service data infrastructure. Data Mesh aims to provide AI/ML teams with easy access to the data products they need, without relying on centralized IT bottlenecks. Imagine a data catalog specifically designed for regulatory reporting. Compliance teams could use this catalog to easily find and access the necessary data for reporting requirements, without having to submit complex data requests to IT. This self-service approach empowers AI/ML teams to experiment and innovate more quickly, accelerating the development and deployment of AI-powered solutions.
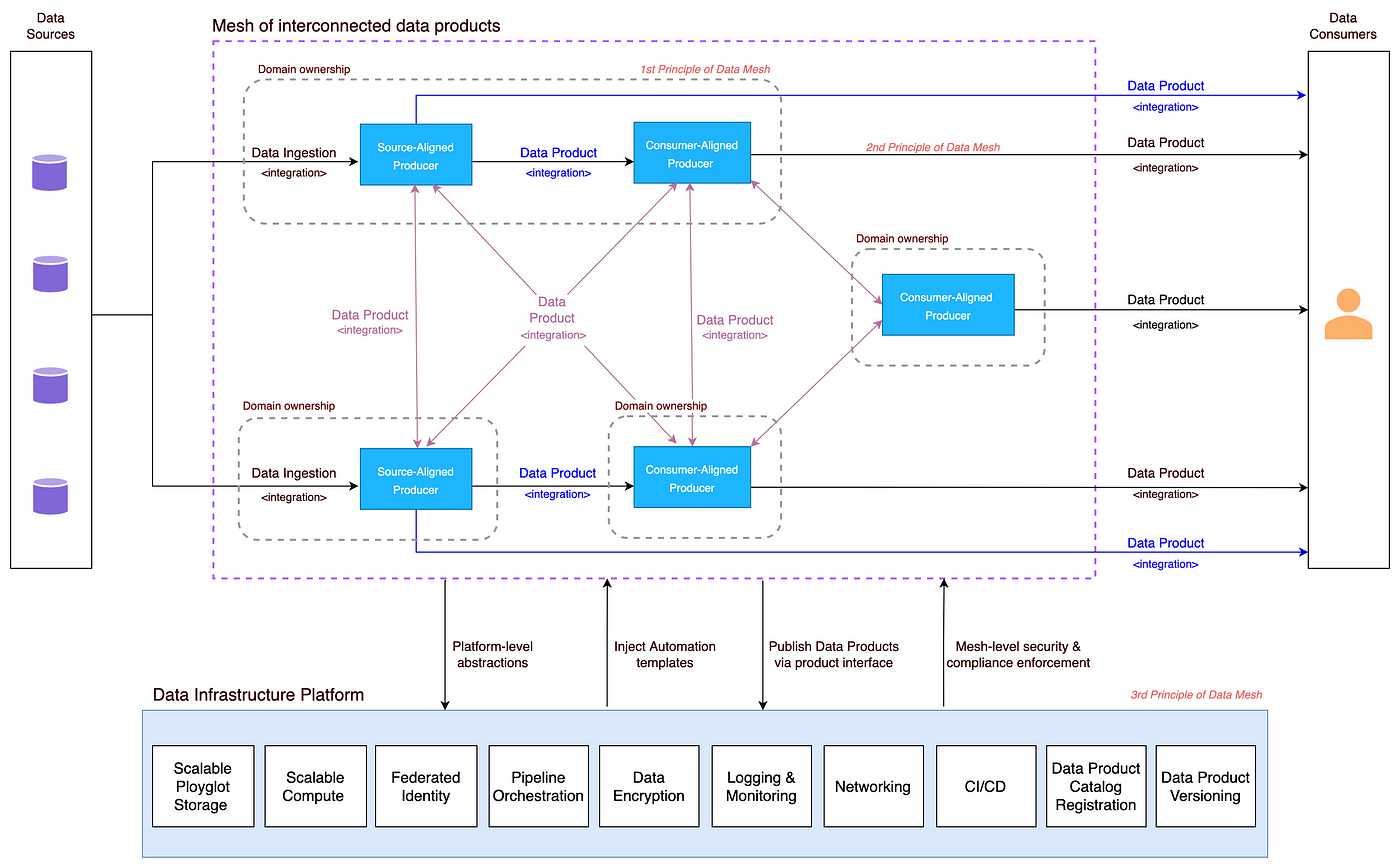
Principle #4 - Federated Governance
Finally, federated governance provides the necessary balance between decentralization and standardization. While domain teams own their data, they must adhere to overarching governance policies related to data quality, security, and privacy. This is particularly important in banking, where regulatory requirements are stringent. For example, a bank might allow agile AI experimentation while simultaneously enforcing strict data privacy and security protocols. Federated governance ensures that data is managed responsibly and ethically, mitigating risks while enabling innovation. This balance is crucial for banks to leverage AI effectively while maintaining compliance and customer trust.

Having a federated model with a team at each domain level can help handle all data governance activities across areas like business glossary and technical and operational metadata resulting in enhanced data quality. Data owners, stewards, data governance experts along with data engineering teams must be a core part of the governance team defined at the domain level. Roles and responsibilities must be divided between different actors both at the domain as well as the central level (see figure below).
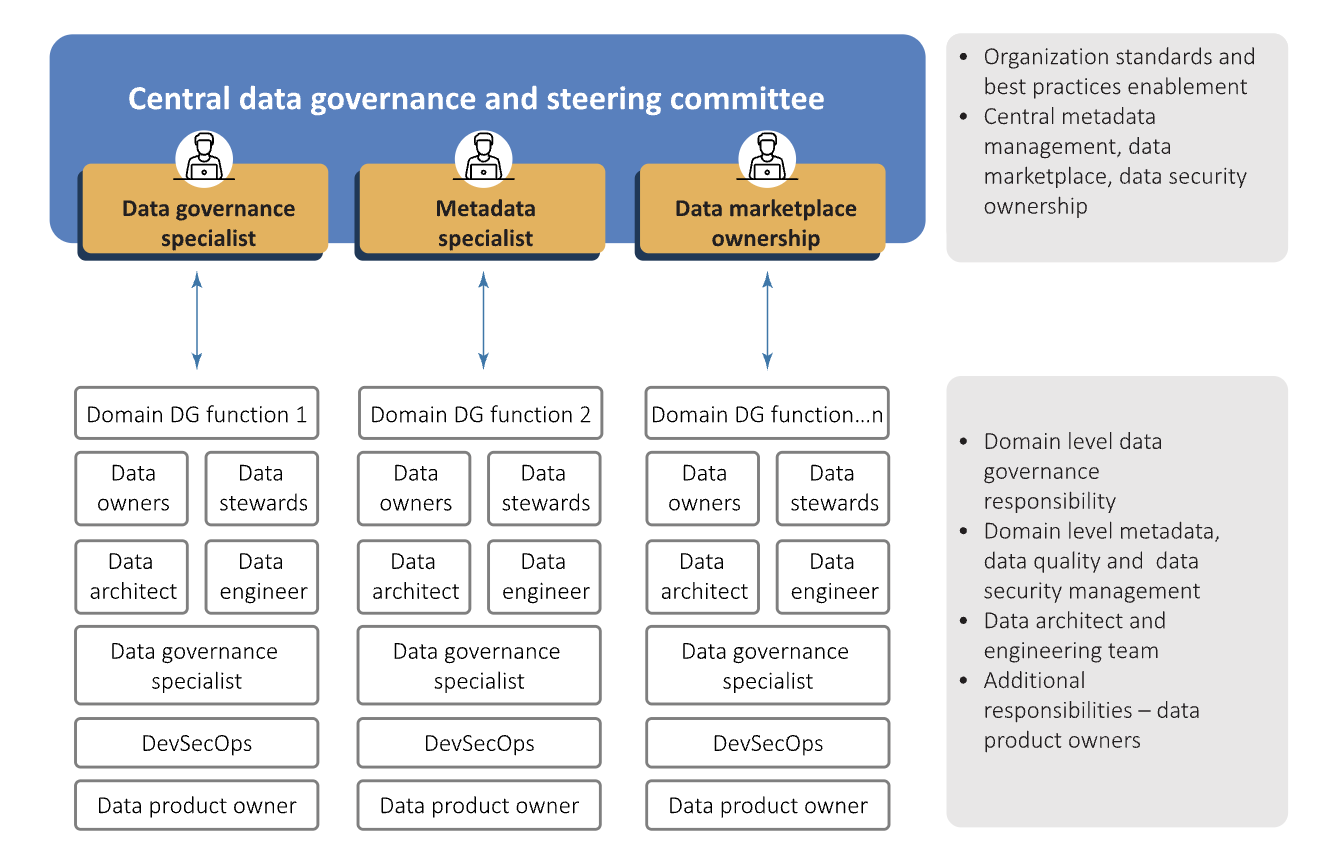
Ownership of data products needs to be established along with data ownership within the realm of data domains. Data product owners must be held responsible for conceptualizing, developing, enhancing, and measuring the usefulness of data products along with improving the quality and richness of the data for internal as well as external ecosystems.
Lessons for a New Data Architecture
The explosion of data and its diverse uses has pushed many companies' data architectures to their limits, making tactical fixes inadequate. A fundamental shift is needed: a flexible data architecture designed for both current needs and future growth. Three key lessons emerge for companies undertaking this transformation. First, architectures must become more decoupled, federated, and service-oriented, similar to a microservices approach. This allows easier data sharing and simplifies data production and consumption for AI/analytics through well-defined APIs. Domain experts can manage their data products, granting secure access to other domains as needed, while the underlying technical complexity remains abstracted. This also allows for a hybrid approach, where some data products reside in traditional warehouses and others in data lakes, optimizing for specific business needs.

Second, the industry is in the early stages of this architectural shift, lacking established standards and protocols. Like the evolution of software architecture, new standards and tools are necessary for data transfer, service definitions, discovery, and registry. We can expect the emergence of open-source projects, community-driven standards, and commercial tooling to address these needs, similar to how Kubernetes and Spinnaker arose for DevOps and microservices. Data vendors must evolve beyond basic data management and analytics, developing new tools for data format conversion, versioning, observability, operations, MLOps, data product composition and governance, and secure data access.
Finally, cost considerations will heavily influence technology choices. Open-source software will continue to play a vital role in data management due to its lower costs and community-driven development. Hyperscalers (such as Amazon Web Services, Microsoft Azure, Google Cloud Platform) will also continue to drive down storage prices and offer innovative serverless and pay-as-you-go data services, making the cloud the center of gravity for data and analytics. The multicloud approach will become increasingly prevalent as organizations seek to avoid vendor lock-in while leveraging the best cloud services available.
Azure Approach to Data Mesh
The simplest deployment pattern for building a data mesh architecture involves one data management landing zone and one data landing zone. The data architecture in such a scenario would look like the following:
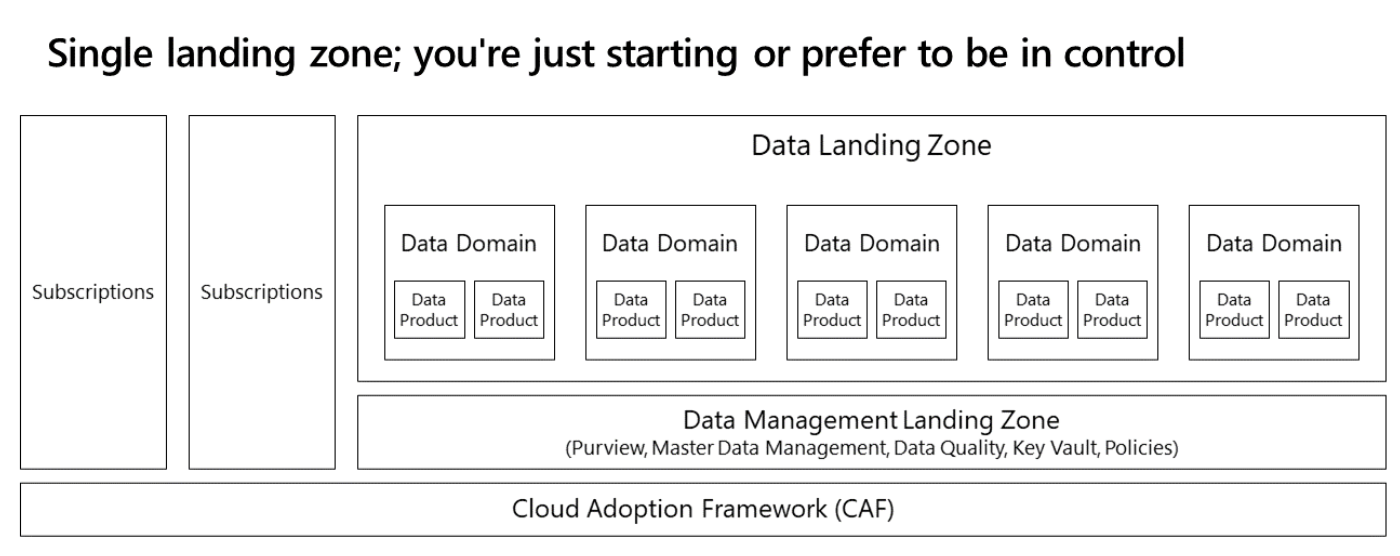
In this model, all your functional data domains reside same data landing zone. A single subscription contains a standard set of services. Resource groups segregate different data domains and data products. Standard data services, like Azure Data Lake Store, Azure Logic Apps and Azure Synapse Analytics, apply to all domains.
In the previous model, we didn't take into account other subscriptions or on-premises applications. You can slightly alter the previous model by adding a source system-aligned landing zone to manage all incoming data. Data onboarding is a difficult process, so having two data landing zones is useful. Onboarding remains one of the most challenging parts of using data at large. Onboarding also often requires extra tools to address integration, because its challenges differ from those of integration. It helps to distinguish between providing data and consuming data.
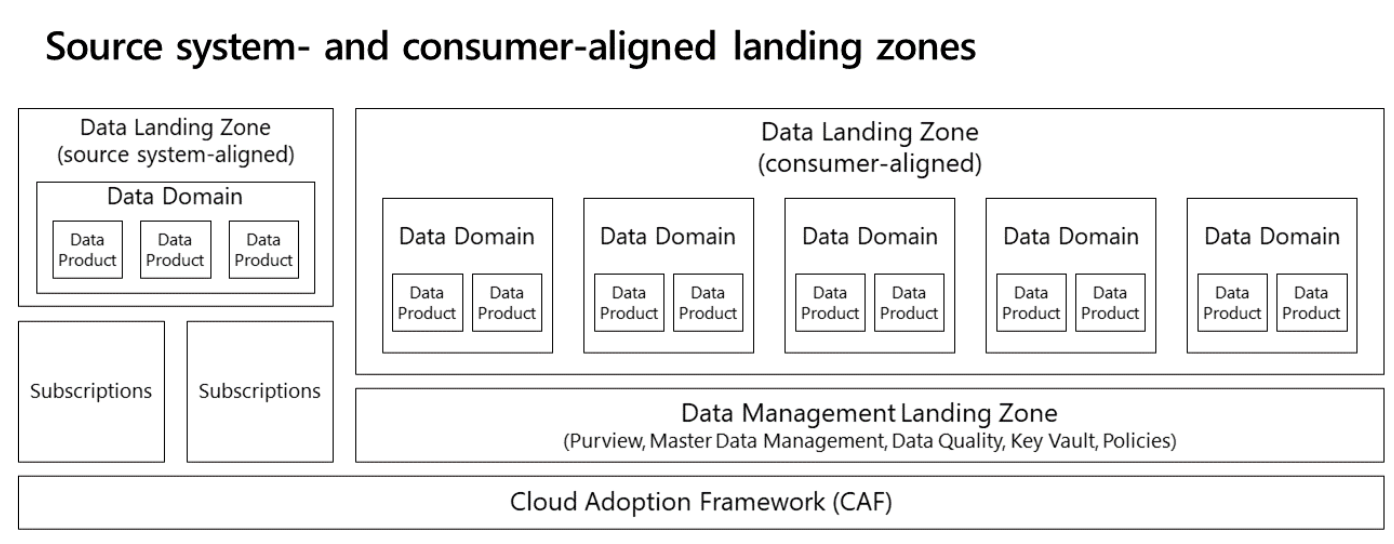
The next deployment option is another iteration of the previous design. This deployment follows a governed mesh topology: data is distributed via a central hub, in which data is partitioned per domain, logically isolated, and not integrated. This model's hub uses its own (domain-agnostic) data landing zone, and can be owned by a central data governance team overseeing which data is distributed to which other domains. The hub also carries services that facilitate data onboarding.
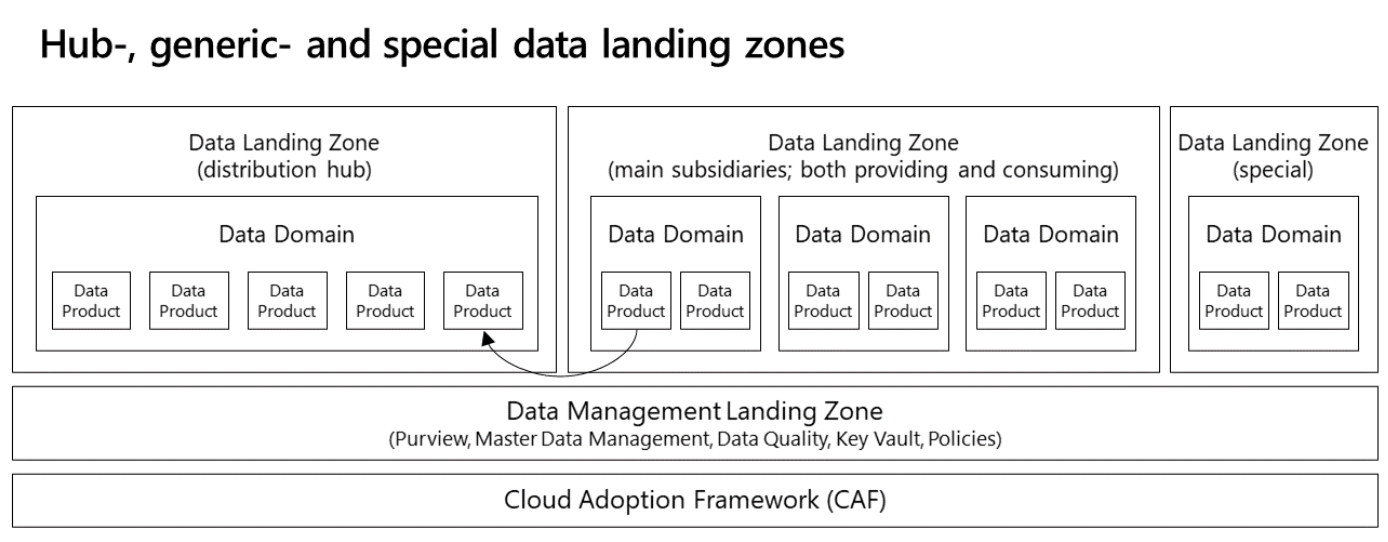
Provisioning multiple data landing zones can help you group functional domains based on cohesion and efficiency for working and sharing data. All your data landing zones adhere to the same auditing and controls, but you can still have flexibility and design changes between different data landing zones.
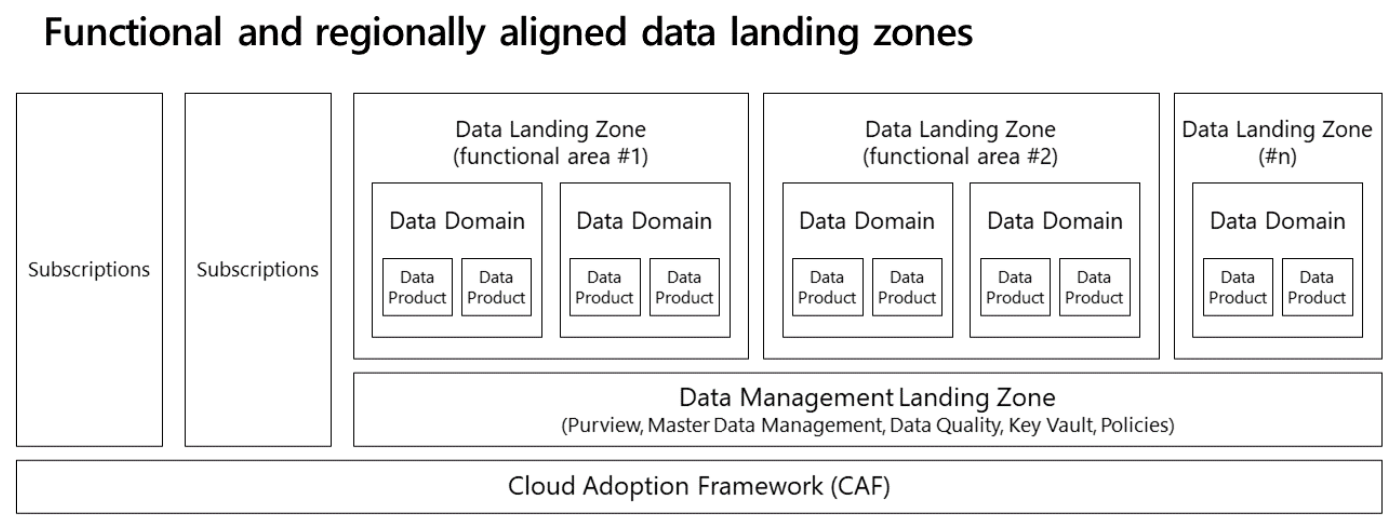
The transition towards data mesh is a cultural shift involving nuances, trade offs and considerations.
Data marketplaces have a strong relationship with metadata. A data marketplace offers data consumers an intuitive, secure, centralized, and standardized data shopping experience. It brings data closer to data analysts and scientists by utilizing the underlying metadata. It also tracks all your data products, which are often stored across a range of data domains.

The domain-driven feature engineering or feature mesh strategy offers a decentralized approach to AI/ML model building in a data mesh setting. The following diagram shows the strategy and how it addresses the four main principles of data mesh.
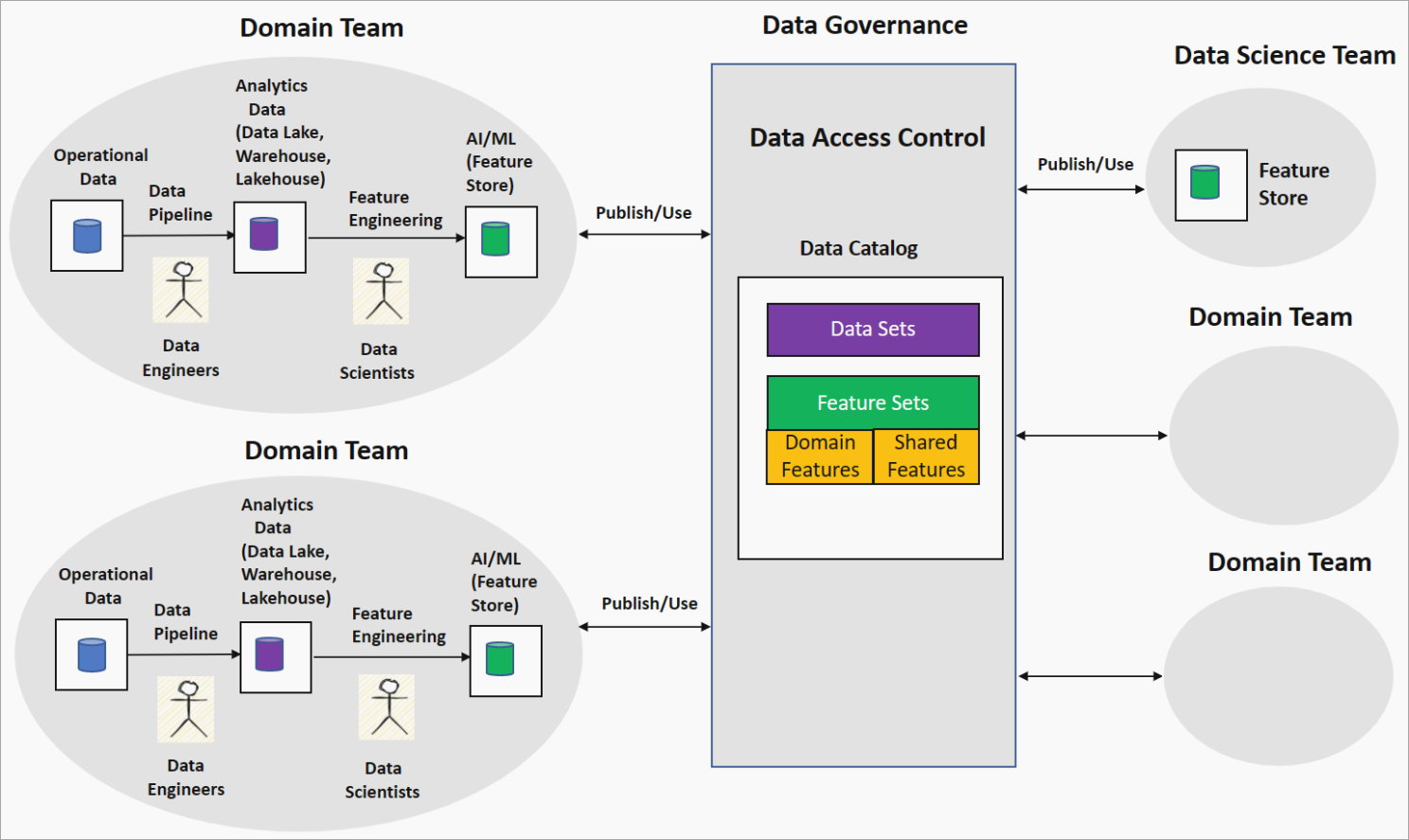
JPMorgan Chase & Fannie Mae run a data mesh
Practical examples offer invaluable learning opportunities, especially when exploring complex concepts like distributed data architectures. Examining real-world implementations can provide deeper insights than theoretical discussions alone.
JPMorgan Chase
Distributed data architectures, designed to address the limitations of traditional monolithic approaches like data warehouses and lakes, operate on several core principles. Data ownership resides with business domains, not a central technical team. Data is treated as a valuable product, not simply an asset. A self-service platform empowers users to add, access, and manage data independently. Finally, governance is decentralized, residing within each domain while adhering to global standards and automated decision-making.

Three very senior leaders at JPMorgan Chase presented about their data mesh journey so far, including moving from on-prem to the cloud, with true honesty about what is working and what is a work-in-progress. A detail post done by Dave Vellante.
Topics included how they are mapping domains to high level products with a number of sub-products and how they are handling a very strict regulatory environment (basically - let's not try to move the really tough stuff to data mesh first). Extensive Q&A with the team as well. Recorded July 8, 2021, hosted by Data Mesh Learning (DML), a vendor-independent resource for learning about data mesh.
Fannie Mae
Fannie Mae store their data in Amazon Redshift data warehouse and S3 Data Lake, and call it Data Lakehouse. All data is registered in a centralised AWS Glue Catalog, which all users can browse to find the data they need: (image source)

To enable a seamless access to data across accounts and business units, we looked at various options to build an architecture that is sustainable and scalable. The data mesh architecture allowed us to keep data of the respective business units in their own accounts, but yet enable a seamless access across the business unit accounts in a secure manner. We reorganized the AWS account structure to have separate accounts for each of the business units wherein, business data and dependent applications were collocated in their respective AWS Accounts.
Each line of business (LOB) creates and maintains their own data sets, registers them as data products in the centralized Glue Data Catalog and shares them (point #1 and #2 in the above diagram). Business applications can consume data from other LOBs by creating a data contract, which is reviewed and approved by the data producer (point #3 and #4 in the above diagram).
In the above diagram, the Contract Registry is hosted on Amazon Dynamo DB. The Access Management layer is built using AWS IAM (Identity & Access management). It provides Just In Time access through IAM session policies using a persona-driven approach. It also provides logging and monitoring.
Data from operational systems are stored in the lakehouse (not the Databricks definition, but Redshift + S3) and the metadata is stored in the centralized data catalogue (see diagram below).

Fannie Mae built a web frontend to facilitate data discovery and data subscription to the Redshift warehouse and S3 lake. The workflow for access request, approval and access provision is fully automated using APIs and AWS CLI, and takes only a few minutes. The business applications can then access the required data in the corresponding lakehouse using Redshift cross-account data sharing (image source).
Azure'z take on banking and data mesh.
Unlocking Business Value with Data Mesh and AI: A CDO/Head of Data Science Perspective in Banking
Let's delve into how Data Mesh and AI intersect to deliver tangible business value in banking, from the perspectives of CDOs and Heads of Data Science:
for Chief Data Officers (CDOs)
From my perspective, I've seen firsthand how data governance and AI have become inextricably linked. Data Mesh offers a compelling solution to the governance challenges we face, especially when it comes to AI. Data Mesh promotes decentralized data ownership, which inherently improves governance. Each domain is responsible for the quality, lineage, and security of its data, making it easier to comply with regulations like GDPR, CCAR, and KYC/AML. For AI, this is crucial. For example, consider KYC/AML compliance. A Data Mesh would ensure that the data used to train an AI model for suspicious activity detection is properly governed within the "Customer" domain. This includes:
- Data Lineage: Tracking the origin and transformation of data used in the AI model, demonstrating compliance to auditors. Example: A metadata tag within the "Customer" data product showing that customer address data was sourced from the core banking system and verified against a third-party database.
- Access Control: Restricting access to sensitive data used in AI models based on roles and responsibilities. Example: Only authorized data scientists and compliance officers can access the training data for the KYC/AML model.
- Data Quality: Ensuring the data used for AI training is accurate, complete, and consistent. Example: Data quality checks within the "Customer" domain ensuring that customer names are consistently formatted and addresses are valid.
AI models are only as good as the data they are trained on. Data Mesh's emphasis on "data as a product" fosters a culture of data quality. When domain teams are responsible for their data products, they are incentivized to maintain high quality. This directly translates to more accurate and reliable AI models. For example, in fraud detection, if the transaction data used to train the AI model is incomplete or inaccurate, the model may fail to identify fraudulent transactions. A well-maintained "Transaction" data product within a Data Mesh would ensure data quality, leading to a more accurate fraud detection model.Data Mesh's self-service data infrastructure and decentralized ownership streamline the process of accessing and preparing data for AI. This significantly reduces the time it takes to develop and deploy AI-powered products and services. For example, consider developing an AI-powered loan approval system. With a Data Mesh, the data scientists can directly access the required data products (e.g., "Customer," "Loan Application," "Credit Score") from their respective domains, rather than waiting for a centralized data team to provide it. This accelerates the development process and allows the bank to bring the AI-powered loan approval system to market faster.for Heads of Data ScienceData Mesh simplifies data discovery and access. Data scientists can easily find and access the data products they need through a data catalog or marketplace. For example, a data scientist building a customer churn prediction model can easily discover and access the "Customer," "Transaction," and "Product Usage" data products through the data catalog. Because these are treated as products, they are well-documented and readily accessible. This eliminates the need for data scientists to spend significant time searching for and requesting data.# Example (Conceptual - Data access would depend on the specific platform)# Assuming a data catalog APIcustomer_data = data_catalog.get_data_product("Customer")transaction_data = data_catalog.get_data_product("Transaction")# ... and so onData Mesh's emphasis on data as a product, with well-defined schemas and metadata, simplifies data preparation for AI/ML. Data scientists can spend less time on data cleaning and transformation and more time on feature engineering and model building. For example, if the "Transaction" data product has a well-defined schema and data quality checks in place, the data scientist can directly use it for feature engineering, such as calculating spending patterns or creating aggregated features.# Example (Conceptual - Feature engineering would be specific to the use case)# Assuming the transaction_data is a Pandas DataFrametransaction_data['transaction_amount'] = transaction_data['amount'].astype(float)transaction_data['day_of_week'] = transaction_data['date'].dt.dayofweek# ... other feature engineering stepsData Mesh's self-service data infrastructure empowers data scientists to build and deploy AI products independently. They don't have to rely on centralized IT teams for data access or infrastructure provisioning. For example, a data scientist building an AI-driven fraud detection system can access the necessary data products, build the model, and deploy it as a service, all without needing extensive IT support. This accelerates the development and deployment cycle and enables faster innovation. They might leverage MLOps platforms that integrate with the Data Mesh to automate model training, deployment, and monitoring.# Example (Conceptual - Model deployment depends on the platform)# Assuming a model serving platformmodel_service = ModelService(model, data_products=["Transaction", "Customer"])model_service.deploy()Data Mesh Implementation in Banking: Key Considerations for AI SuccessImplementing a Data Mesh in a bank, especially one geared towards AI success, isnt just a technical undertaking; it demands significant organizational and cultural change, careful technology choices, and robust governance. Lets unpack these key considerations.Organizational and Cultural Shifts for Data & AI CollaborationBanks are traditionally siloed, and data teams, AI/ML teams, and business stakeholders often operate in isolation. This hinders effective data utilization for AI. Data Mesh requires breaking down these silos and fostering a culture of collaboration. Specifically, we need to address:
- Bridging the Gap: Data teams need to understand the needs of AI/ML teams, and vice-versa. We need joint workshops, shared projects, and cross-functional teams to build mutual understanding and trust. Business stakeholders must be actively involved in defining AI use cases and prioritizing data products.
- Shifting Mindsets: Data Mesh requires a shift from a centralized "data hoarding" mentality to a decentralized "data sharing" mindset. Domain teams must embrace ownership of their data products and understand their responsibility in making them discoverable, accessible, and usable for others, including AI/ML teams.
- Incentivizing Collaboration: Performance metrics and incentives should be aligned to encourage collaboration and data sharing. For example, domain teams could be recognized for the impact their data products have on AI initiatives.
Technology and Infrastructure: Building the Foundation for AI in BankingA successful Data Mesh implementation requires a robust and flexible technology stack. In banking, this often means integrating with existing infrastructure while embracing modern technologies:
- Integrating with Legacy: Core banking systems and data warehouses are still critical components of a bank's IT landscape. Data Mesh must be able to integrate with these systems to access valuable historical data. This might involve APIs, data virtualization, or change data capture (CDC) mechanisms.
- Embracing Cloud-Native: Cloud platforms offer the scalability, flexibility, and cost-effectiveness needed to support Data Mesh and AI initiatives. Cloud-native architectures, including microservices, containers, and serverless computing, enable agile development and deployment of data products and AI models.
- API-Driven Connectivity: APIs are the glue that holds a Data Mesh together. Well-defined APIs enable data products to be easily discovered and accessed by other domains and AI/ML teams. This promotes interoperability and reduces data silos.
- Orchestration Layer: This is where the rubber meets the road. Tools like Customer Lifetime Orchestrator (CLO) play a crucial role in connecting data insights to banking-specific actions. CLO can consume data products from the Data Mesh to personalize customer interactions, automate marketing campaigns, and optimize customer lifetime value. It's the bridge between data and action.
Governance and Security in the Age of AI in BankingGovernance and security are paramount in banking, especially with the rise of AI. Data Mesh must address these concerns:
- Data Privacy: Banks handle highly sensitive customer data. Data Mesh must incorporate robust data privacy mechanisms, such as anonymization, pseudonymization, and differential privacy, to ensure compliance with regulations like GDPR and CCPA. This is particularly important for AI models trained on customer data.
- Regulatory Compliance: Banking regulations are complex and constantly evolving. Data Mesh must facilitate compliance by providing clear data lineage, audit trails, and access controls. This is crucial for AI applications used in areas like risk management and regulatory reporting.
- Model Risk Management (MRM): AI models used in banking must be rigorously validated and monitored to ensure they are accurate, reliable, and unbiased. Data Mesh can support MRM by providing access to the data used to train the models and enabling transparency in model development.
- Decentralized Responsibility, Centralized Oversight: While domains own their data, there needs to be centralized oversight to ensure compliance with global standards and policies. This can be achieved through a federated governance model that balances autonomy with control.
Data Mesh offers a powerful framework for banks to unlock the full potential of their data and AI initiatives. However, successful implementation requires careful attention to organizational and cultural factors, technology choices, and governance. By addressing these key considerations, banks can build a robust and scalable Data Mesh that empowers them to become truly data-driven and AI-first.Orchestrating Customer Value: The Role of CLO in the Banking Data MeshIn a Data Mesh architecture, data products are the building blocks, but it's the orchestration layer that brings them to life and drives tangible business outcomes. This is where Customer Lifetime Orchestrator (CLO) solutions, like Backbase's, play a critical role. CLO acts as the conductor of the data orchestra, integrating with the Data Mesh to consume data products and translate them into meaningful actions that enhance customer value.CLO integrates seamlessly with the Data Mesh by acting as a consumer of the various data products residing within the mesh. For example, a "Customer Profile" data product might contain demographic information, transaction history, and product holdings. A "Product Catalog" data product would detail the bank's offerings, including loan terms, mortgage rates, and investment options. A "Customer Interaction" data product would capture customer interactions across channels, such as website visits, mobile app usage, and call center logs. CLO ingests these data products, combining and enriching them to create a holistic view of each customer. This enriched data fuels personalized experiences and maximizes customer lifetime value (CLV).CLO empowers banks to orchestrate data and insights to personalize banking experiences in several ways:
- Improved Customer Engagement: Imagine a customer browsing mortgage rates on the bank's website. CLO can access the "Customer Profile" and "Product Catalog" data products to identify the customer's pre-approved loan amount and offer personalized mortgage options with competitive rates. This proactive approach increases the likelihood of conversion and strengthens customer engagement. Backbase's CLO, for example, can trigger personalized notifications within the customer's banking app, offering tailored mortgage advice based on their browsing behavior and financial profile.
- Increased Product Adoption: CLO can analyze customer data to identify unmet needs and recommend relevant financial products. For example, a customer who recently started a family might be interested in life insurance or education savings plans. CLO can leverage the "Customer Profile" and "Product Catalog" data products to identify this need and proactively offer tailored financial advice and product recommendations. Backbase's platform allows banks to create personalized journeys, guiding customers towards the right products based on their life events and financial goals.
- Reduced Churn: By analyzing customer interaction data and identifying patterns of disengagement, CLO can trigger proactive interventions to reduce churn. For example, if a customer hasn't logged into their banking app for several weeks, CLO can trigger a personalized email or push notification offering assistance or highlighting new features. Backbase's CLO can even integrate with customer service platforms, alerting agents to potential churn risks and empowering them to proactively reach out to at-risk customers.
Here are some more specific examples of how CLO, within a Data Mesh context, can drive value:
- Personalized Loan Offers: CLO can combine data from the "Customer Profile," "Transaction History," and "Credit Score" data products to generate personalized loan offers with tailored interest rates and repayment terms.
- Tailored Financial Advice: CLO can analyze customer spending patterns, investment goals, and risk tolerance (all residing in relevant data products) to provide personalized financial advice and investment recommendations.
- Proactive Customer Support: CLO can identify customers experiencing difficulties with online banking services by analyzing their interaction data. This allows the bank to proactively offer assistance through chat, email, or phone, improving customer satisfaction and reducing support costs.
In essence, CLO acts as the intelligent layer that connects the dots between data and action. By integrating with a Data Mesh, CLO empowers banks to move beyond simply collecting data to actively using it to personalize customer experiences, drive revenue growth, and build stronger customer relationships. It's about transforming data into actionable insights that create value for both the bank and its customers.The Future of Banking: Data Mesh, AI, and the Rise of the Data-Driven BankThe future of banking is inextricably linked to data and AI. Data Mesh and AI are not just incremental improvements; they represent a fundamental transformation in how banks operate, compete, and serve their customers. The convergence of these technologies is ushering in the era of the data-driven bank, a future where personalized experiences, optimized operations, and continuous innovation are the norm.Data Mesh and AI have the potential to reshape the banking industry in profound ways:
- Hyper-Personalization: Imagine a world where every customer interaction is tailored to their specific needs and preferences. Data Mesh provides the foundation for this level of personalization by making relevant data readily accessible. AI, powered by this data, can then anticipate customer needs, offer proactive advice, and deliver truly personalized experiences across all touchpoints. This could range from personalized loan offers and investment recommendations to proactive fraud alerts and tailored financial education.
- Intelligent Automation: Many banking processes are ripe for automation. AI, fueled by data from the Data Mesh, can automate tasks such as loan processing, KYC/AML checks, and customer support. This not only reduces costs and improves efficiency but also frees up human employees to focus on more complex and strategic tasks.
- Real-Time Insights and Decision-Making: Real-time data streaming, combined with AI, enables banks to make faster and more informed decisions. For example, real-time transaction data can be used to detect fraudulent activity as it happens, allowing for immediate intervention. Real-time market data can inform trading decisions and risk management strategies.
- New Product and Service Innovation: Data Mesh and AI can unlock entirely new possibilities for product and service innovation. By understanding customer needs and preferences at a granular level, banks can develop innovative financial products and services that cater to specific segments. AI can also be used to develop personalized financial advice tools and robo-advisors.
- Enhanced Risk Management: AI models, trained on comprehensive data sets from the Data Mesh, can improve risk management in areas such as credit scoring, fraud detection, and regulatory compliance. These models can identify patterns and anomalies that would be difficult for humans to detect, leading to more accurate risk assessments and better mitigation strategies.
Several emerging trends and technologies complement Data Mesh and AI, further accelerating the transformation of banking:
- Cloud Computing: Cloud platforms provide the scalable and cost-effective infrastructure needed to support Data Mesh and AI initiatives. Cloud-native architectures enable agile development and deployment of data products and AI models.
- Engagement Banking Platforms: Engagement Banking Platform like what Backbase offers, empowers banks to modernize their offerings, improve customer satisfaction, and deliver a consistent experience across all digital touch points. Also make it ready for AI Agents and Data Mesh.
- Real-Time Data Streaming: Technologies like Apache Kafka enable the capture and processing of data in real time. This is crucial for applications that require immediate insights and decision-making, such as fraud detection and personalized offers.
- MLOps: MLOps platforms automate and streamline the entire machine learning lifecycle, from data preparation and model training to deployment and monitoring. This enables banks to develop and deploy AI models more quickly and efficiently.
- Federated Learning: This technique allows AI models to be trained on decentralized data sets without the data ever leaving its source. This is particularly relevant for banking, where data privacy is paramount.
- Explainable AI (XAI): As AI becomes more prevalent in banking, it's crucial to understand how AI models make decisions. XAI techniques provide transparency into model behavior, which is essential for building trust and ensuring regulatory compliance.
The data-driven bank of the future will be a truly customer-centric organization. AI will be woven into every aspect of the customer journey, from personalized onboarding experiences to proactive financial advice. Operations will be optimized through intelligent automation and real-time insights. Innovation will be continuous, with banks constantly developing new products and services to meet evolving customer needs. Data Mesh and AI will not only transform individual banks but also reshape the entire financial ecosystem, leading to greater efficiency, increased competition, and ultimately, better outcomes for customers. This future is not far off; the journey to becoming a data-driven bank has already begun.